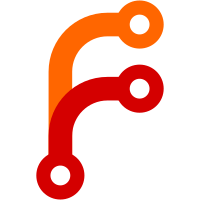
This PR adds the docs app back into the tldraw monorepo. ## Deploying We'll want to update our deploy script to update the SOURCE_SHA to the newest release sha... and then deploy the docs pulling api.json files from that release. We _could_ update the docs on every push to main, but we don't have to unless something has changed. Right now there's no automated deployments from this repo. ## Side effects To make this one work, I needed to update the lock file. This might be ok (new year new lock file), and everything builds as expected, though we may want to spend some time with our scripts to be sure that things are all good. I also updated our prettier installation, which decided to add trailing commas to every generic type. Which is, I suppose, [correct behavior](https://github.com/prettier/prettier-vscode/issues/955)? But that caused diffs in every file, which is unfortunate. ### Change Type - [x] `internal` — Any other changes that don't affect the published package[^2]
241 lines
7.2 KiB
TypeScript
241 lines
7.2 KiB
TypeScript
import { connect } from '@/scripts/functions/connect'
|
|
import { Article, ArticleHeading, ArticleHeadings } from '@/types/content-types'
|
|
import { config } from 'dotenv'
|
|
import OpenAI from 'openai'
|
|
import path from 'path'
|
|
import { LocalIndex } from 'vectra'
|
|
import { nicelog } from './nicelog'
|
|
|
|
config()
|
|
|
|
const MAX_ARTICLES = Infinity
|
|
const INCLUDE_API_CONTENT = true
|
|
const INCLUDE_CONTENT = true
|
|
|
|
const index = new LocalIndex(path.join(process.cwd(), 'utils', 'vector-db'))
|
|
|
|
const openai = new OpenAI({
|
|
apiKey: process.env.OPENAI_KEY,
|
|
})
|
|
|
|
export class ContentVectorDatabase {
|
|
index: LocalIndex
|
|
api: OpenAI
|
|
|
|
constructor(opts = {} as { index: LocalIndex; api: OpenAI }) {
|
|
this.index = opts.index
|
|
this.api = opts.api
|
|
}
|
|
|
|
/**
|
|
* Get a vector from a piece of text from openai.
|
|
*
|
|
* @param text The text to get a vector for.
|
|
*
|
|
* @returns The vector.
|
|
*/
|
|
async getVectorEmbeddings(inputs: string[]) {
|
|
const response = await this.api.embeddings.create({
|
|
model: 'text-embedding-ada-002',
|
|
input: inputs,
|
|
})
|
|
return response.data.map((d) => d.embedding)
|
|
}
|
|
|
|
async addHeadingToIndex(article: Article, heading: ArticleHeading) {
|
|
const id = `${article.id}#${heading.slug}`
|
|
|
|
// Skip headings that are already present
|
|
const hash = this.getHashForString(heading.title + heading.slug)
|
|
const existingItem = await this.index.getItem(id)
|
|
if (existingItem) {
|
|
if (existingItem.metadata.hash === hash) {
|
|
nicelog(`Skipping heading ${id} (already present)`)
|
|
return
|
|
}
|
|
await this.index.deleteItem(id)
|
|
}
|
|
|
|
nicelog(`Adding headers for ${article.title}#${heading.title}`)
|
|
const vectors = await this.getVectorEmbeddings([article.title + '#' + heading.title])
|
|
this.index.insertItem({
|
|
id,
|
|
vector: vectors[0],
|
|
metadata: { type: 'heading', articleId: article.id, slug: heading.slug, hash },
|
|
})
|
|
}
|
|
|
|
/**
|
|
* Add a text item to the index.
|
|
*
|
|
* @param text The text to add to the index.
|
|
*
|
|
* @returns The index item.
|
|
*/
|
|
async addArticleToIndex(article: Article, headings: ArticleHeadings) {
|
|
// This is the content that we'll create the embedding for
|
|
let contentToVectorize: string
|
|
|
|
if (article.sectionId === 'gen') {
|
|
// For API docs, we'll just use the title, description, and members as the content.
|
|
// We'll also add a note that the content was generated from the API docs, hopefully
|
|
// so that the embedding better reflects searches for api docs.
|
|
contentToVectorize = `Title: ${article.title}\nPackage: @tldraw/${article.categoryId}\nDescription: ${article.description}\nMembers:${article.keywords}\n\n(content generated from API docs)`
|
|
} else {
|
|
// The content is the raw markdown content, which includes all the markdown
|
|
// headings and annotations, though none of the frontmatter. We'll add the
|
|
// frontmatter information again manually. We may need to also add some information
|
|
// about how "important" this article is, relative to related docs or headings.
|
|
contentToVectorize = `Title: ${article.title}\nDescription: ${article.description}\nKeywords:${article.keywords}\nMarkdown:\n${article.content}`
|
|
}
|
|
|
|
if (headings.length) {
|
|
for (const heading of headings) {
|
|
await this.addHeadingToIndex(article, heading)
|
|
}
|
|
}
|
|
|
|
// Generate a hash based on the content that we'd be vectorizing
|
|
const hash = this.getHashForString(contentToVectorize)
|
|
|
|
// Create chunks from the content; openAI has a limit of 500 tokens per request
|
|
const chunksToAdd: string[] = []
|
|
const chunkSize = 500
|
|
for (let i = 0; i < contentToVectorize.length; i += chunkSize) {
|
|
const chunk = contentToVectorize.slice(i, i + chunkSize)
|
|
chunksToAdd.push(chunk)
|
|
}
|
|
|
|
// Is there already an item with this id?
|
|
const existingItem = await this.index.getItem(article.id + '_0')
|
|
|
|
if (existingItem) {
|
|
// ...and if the existing item matches our hash, we can skip it
|
|
if (existingItem.metadata.hash === hash) {
|
|
nicelog(`Skipping ${article.id} (already present)`)
|
|
return
|
|
}
|
|
|
|
// ...otherwise, delete all the chunks so that we can add a new one.
|
|
for (let i = 0; i < chunksToAdd.length; i++) {
|
|
await this.index.deleteItem(article.id + '_' + i)
|
|
}
|
|
}
|
|
|
|
// Add chunks to index
|
|
nicelog(`Adding article ${article.title} (${chunksToAdd.length} chunks)`)
|
|
|
|
// Get an embedding / vector for all of the chunks
|
|
const vectors = await this.getVectorEmbeddings(chunksToAdd)
|
|
|
|
for (let i = 0; i < vectors.length; i++) {
|
|
const vector = vectors[i]
|
|
// Add the article item to the index (include the hash as metadata)
|
|
await this.index.insertItem({
|
|
id: article.id + '_' + i,
|
|
vector: vector,
|
|
metadata: { type: 'article', articleId: article.id, hash },
|
|
})
|
|
}
|
|
|
|
// Sleep for 50ms or so to avoid rate limiting
|
|
await new Promise((r) => setTimeout(r, 35))
|
|
|
|
return
|
|
}
|
|
|
|
/**
|
|
* Query an item using our index.
|
|
*
|
|
* @param text The text to query.
|
|
*
|
|
* @returns The query results.
|
|
*/
|
|
async query(text: string, limit = 5) {
|
|
const vector = await this.getVectorEmbeddings([text])
|
|
const results = await this.index.queryItems(vector[0], limit)
|
|
const output: (
|
|
| { id: string; type: 'article'; score: number }
|
|
| { id: string; type: 'heading'; slug: string; score: number }
|
|
)[] = []
|
|
const visited = new Set<string>()
|
|
for (const result of results) {
|
|
const id = result.item.metadata.articleId as string
|
|
const type = result.item.metadata.type as 'article' | 'heading'
|
|
if (type === 'heading') {
|
|
const slug = result.item.metadata.slug as string
|
|
output.push({ id, type, slug, score: result.score })
|
|
} else {
|
|
// multiple chunks may have been returned
|
|
if (visited.has(id)) continue
|
|
output.push({ id, type, score: result.score })
|
|
visited.add(id)
|
|
}
|
|
}
|
|
return output
|
|
}
|
|
|
|
/**
|
|
* Hash a string using the FNV-1a algorithm.
|
|
*
|
|
* @public
|
|
*/
|
|
getHashForString(string: string) {
|
|
let hash = 0
|
|
for (let i = 0; i < string.length; i++) {
|
|
hash = (hash << 5) - hash + string.charCodeAt(i)
|
|
hash |= 0 // Convert to 32bit integer
|
|
}
|
|
return hash + ''
|
|
}
|
|
}
|
|
|
|
let _cvdb: ContentVectorDatabase
|
|
|
|
export async function getVectorDb(
|
|
opts = {} as {
|
|
updateContent?: boolean
|
|
rebuildIndex?: boolean
|
|
}
|
|
) {
|
|
if (_cvdb) {
|
|
return _cvdb
|
|
}
|
|
|
|
if (opts.rebuildIndex || !(await index.isIndexCreated())) {
|
|
await index.createIndex({ deleteIfExists: opts.rebuildIndex, version: 1 })
|
|
}
|
|
|
|
_cvdb = new ContentVectorDatabase({ api: openai, index })
|
|
|
|
if (opts.updateContent || opts.rebuildIndex) {
|
|
nicelog(`Rebuilding index`)
|
|
const db = await connect({ reset: false })
|
|
|
|
nicelog(`Getting articles`)
|
|
const articles =
|
|
INCLUDE_API_CONTENT && INCLUDE_CONTENT
|
|
? await db.all('SELECT * FROM articles')
|
|
: INCLUDE_API_CONTENT
|
|
? await db.all('SELECT * FROM articles WHERE articles.sectionId = ?', 'gen')
|
|
: await db.all('SELECT * FROM articles WHERE articles.sectionId != ?', 'gen')
|
|
|
|
nicelog(`Adding articles to index`)
|
|
const max = Math.min(articles.length, MAX_ARTICLES)
|
|
for (let i = 0; i < max; i++) {
|
|
const article = articles[i]
|
|
const headings = await db.all(
|
|
'SELECT * FROM headings WHERE articleId = ? AND slug NOT IN (?, ?, ?, ?)',
|
|
article.id,
|
|
'constructor',
|
|
'properties',
|
|
'example',
|
|
'methods'
|
|
)
|
|
nicelog(`Adding article ${article.id} to index (${i} of ${max})`)
|
|
await _cvdb.addArticleToIndex(article, headings)
|
|
}
|
|
}
|
|
|
|
return _cvdb
|
|
}
|